New year, new site
..go
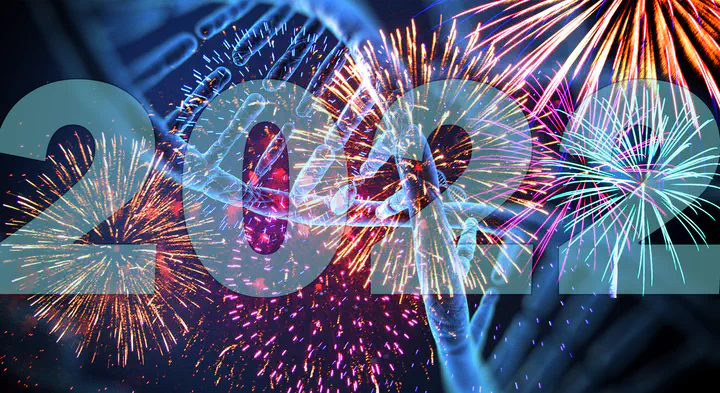
With my recent involvement with the UK Reproducibility Network, and continued use of GitHub for code sharing, I thought it was long past due that I create a quick site to provide access to the code, analysis and associated data for the projects I am working with. The Wellcome Trust funds my position at the Institute of Ophthalmology (UCL) in the Ocular Genomics and Therapeutics lab of Professor Mariya Mosajee, and with the Trust’s supportive attitude to open research I will document some of the projects and code that we are currently involved with.
# Welcome
return("Hello World")
require(stats)
#' Compute different averages
#'
#' @param x \code{numeric} vector of sample data
#' @param type \code{character} vector of length 1 specifying the average type
#' @return \code{centre} returns the sample average according to the chosen method.
#' @examples
#' centre(rcauchy(10), "mean")
#' @export
centre <- function(x, type) {
switch(type,
mean = mean(x),
median = median(x),
trimmed = mean(x, trim = .1))
}
x <- rcauchy(10)
centre(x, "mean")
library(ggplot2)
models <- tibble::tribble(
~model_name, ~ formula,
"length-width", Sepal.Length ~ Petal.Width + Petal.Length,
"interaction", Sepal.Length ~ Petal.Width * Petal.Length
)
iris %>%
nest_by(Species) %>%
left_join(models, by = character()) %>%
rowwise(Species, model_name) %>%
mutate(model = list(lm(formula, data = data))) %>%
summarise(broom::glance(model))
#> `summarise()` regrouping output by 'Species', 'model_name' (override with `.groups` argument)
#> # A tibble: 6 x 13
#> # Groups: Species, model_name [6]
#> Species model_name r.squared adj.r.squared sigma statistic p.value df
#> <fct> <chr> <dbl> <dbl> <dbl> <dbl> <dbl> <int>
#> 1 setosa length-wi… 0.112 0.0739 0.339 2.96 6.18e- 2 3
#> 2 setosa interacti… 0.133 0.0760 0.339 2.34 8.54e- 2 4
#> 3 versic… length-wi… 0.574 0.556 0.344 31.7 1.92e- 9 3
#> 4 versic… interacti… 0.577 0.549 0.347 20.9 1.11e- 8 4
#> 5 virgin… length-wi… 0.747 0.736 0.327 69.3 9.50e-15 3
#> 6 virgin… interacti… 0.757 0.741 0.323 47.8 3.54e-14 4
#> # … with 5 more variables: logLik <dbl>, AIC <dbl>, BIC <dbl>, deviance <dbl>,
#> # df.residual <int>